The AI That Runs the World
- Kieren Sharma
- Mar 27, 2024
- 4 min read
Updated: Feb 28
In this episode, we dive into the pervasive world of recommendation algorithms and explore how they are increasingly shaping our daily lives. From the moment we wake up to the time we go to sleep, these AI systems are constantly at work, influencing our choices and experiences.
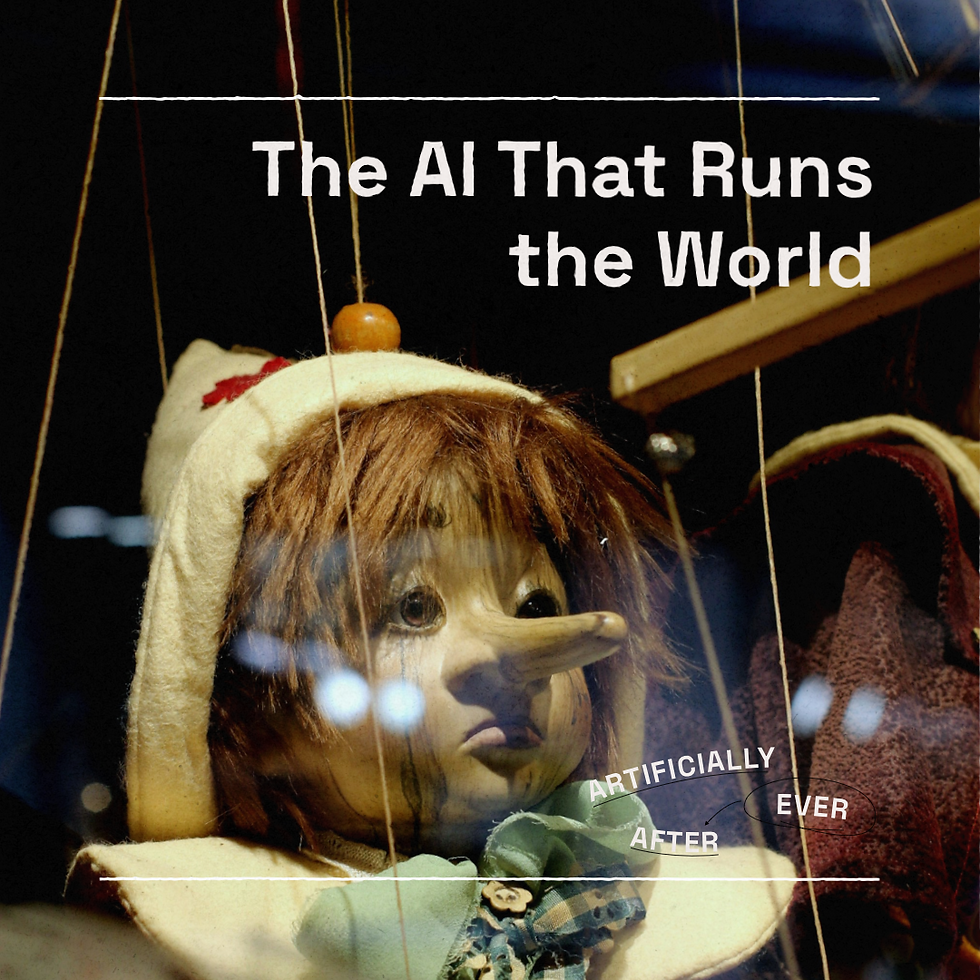
What are Recommendation Algorithms?
Recommendation systems are automated tools designed to suggest the best items or content based on an individual's preferences and past behaviours. They aim to solve the problem of information overload by filtering the vast amount of data available and presenting users with what they are most likely to be interested in.
The first-ever recommendation system, known as the Grundy system, was created in 1979 and it worked using a predefined set of rules to suggest books within a library. But today, most recommendation systems use sophisticated deep-learning methods to learn patterns from vast amounts of data.
There are two primary ways of suggesting content:
User-based filtering: Recommends items based on the preferences of similar users. For instance, if a system identifies that cyclists tend to like non-alcoholic beer, it will recommend non-alcoholic beer to new cyclists.
Item-based filtering: Recommends items similar to those a user has previously shown interest in. For example, if you watch a lot of rom-coms on Netflix, the system will recommend other movies in that genre.
Most of today's recommendation systems combine these two methods into a hybrid system.
Where are They Used?
Recommendation algorithms are used in various applications, and some examples we identified include:
Music Streaming: Spotify uses algorithms to recommend new music and curate playlists.
Search Engines: Google's search results are tailored based on your past activity.
Predictive Text: Autocomplete features on your phone use algorithms to predict the next word you want to type.
Social Media: Platforms like Facebook, Instagram and LinkedIn use algorithms to curate the content that you see on your feeds.
Email: Email spam filters use algorithms to prioritise and filter your emails.
News: News apps like Apple News and BBC News use recommendation algorithms to suggest relevant news stories.
Entertainment: Streaming services like Netflix use algorithms to recommend movies and shows based on your viewing history.
Navigation: Mapping apps like Google Maps use algorithms to suggest routes and locations.
How Are They Trained?
Recommendation algorithms are trained using historical data on user interactions. This data includes what users click on, how long they look at content, what they share, and how they rate products. The systems then use this data to identify patterns and predict what a user will do next. The more data the system gathers, the better it gets at understanding your behaviour. For example, short video platforms like TikTok have some of the most advanced algorithms because they have access to so much data on user behaviour.
Are They Serving Us or Persuading Us?
While recommendation systems are designed to help us navigate the vast amount of information available online, we discussed whether these systems are actually serving our best interests, or are they simply persuading us to spend more time on these platforms.
It's important to remember that these free platforms generate revenue through advertising. Thus, their primary goal is to maximize user engagement, which often means maximizing clicks and time spent on the platform. For example, 60% of TikTok users spend an average of 10 hours per week on the app. These systems learn from user behaviour, and this can lead to some concerning trends. For example:
Polarisation: Recommendation systems can create echo chambers where users are only exposed to information that confirms their existing beliefs, leading to significant polarisation.
Negative News: People are more attracted to negative news and morally controversial content, so this type of content gets circulated more, and this is what the algorithms pick up as interesting.
Key Considerations for the Future
The episode concludes with a number of key points that we should consider as users and citizens interacting with these platforms:
Transparency: It is important that users have the ability to see how these systems are working and what data they are using from us. For example, X, previously Twitter, open-sourced their recommendation system, making the algorithm available for anyone to see.
Fairness: These algorithms must be designed to mitigate bias and ensure equitable outcomes for all users.
Privacy: It is vital that personal information is anonymised and that people have some control over their data.
Free Will: Recommendation systems can influence choices, and we must consider if these systems are serving us or persuading us. We mentioned a story where the US supermarket Target predicted a teenager's pregnancy before the teenager's father even knew.
Regulation: As these systems become ever more powerful, it is important that governments put regulations in place to ensure that these systems are safe and beneficial to society. This includes greater transparency, user control, and restrictions on how much data a company can collect and sell.
The key message is that recommendation algorithms are a vital part of how we interact with the digital world, and they are not going away. We need to be aware of the impact that they have on our daily lives and make sure that their development and application is done in a responsible way.
If you enjoyed reading, don’t forget to subscribe to our newsletter for more, share it with a friend or family member, and let us know your thoughts—whether it’s feedback, future topics, or guest ideas, we’d love to hear from you!
Comments