An AI a Day Keeps the Doctor Away
- Kieren Sharma
- Apr 26, 2024
- 4 min read
Updated: Feb 28
In this episode, we explored the exciting and complex world of artificial intelligence in healthcare. We looked at the history of AI in medicine, current applications, ethical considerations and future possibilities.

A Look Back
The use of AI in healthcare is not a recent development. As early as 1971, computers were employed to automate medical tasks, such as diagnosing symptoms. By 1976, AI systems were developed to recommend antibiotic treatments for bacterial pathogens. The early 2000s marked a significant turning point with the Human Genome Project and the adoption of high-throughput measurement techniques, which generated vast amounts of data. By 2015, data-driven chatbots like Pharmabot were being utilised to provide information on medicines. In 2017, the US Food and Drug Administration (FDA) approved the first AI-powered tool for clinical settings, which analysed heart MRI scans using AI trained on big data. A major breakthrough came in 2020 with DeepMind’s AlphaFold, which solved the long-standing challenge of protein folding prediction, unlocking new possibilities for understanding the fundamental components of life and fighting disease.
Current Applications of AI in Healthcare
AI is currently being used in various ways to improve healthcare:
Harvard’s School of Public Health estimates that using AI to assist with diagnoses may reduce treatment costs by up to 50% and improve health outcomes by 40%.
Diagnosis and Treatment: AI can analyse medical scans (X-rays, MRIs, etc.) to detect diseases like cancer and heart conditions, sometimes spotting subtle patterns that humans might miss. For example, AI can identify those with a high risk of fatal heart disease up to five years in advance. AI is also being used to help prevent blindness in people with diabetes by predicting diabetic retinopathy.
Patient Engagement: AI chatbots can help improve communication between healthcare professionals and patients, bridge language barriers, and assist with medication adherence.
Administrative Activities: AI can automate administrative tasks, freeing up healthcare professionals to focus on patient care.
Drug Discovery and Design: AI is being used to speed up the drug development process, identifying new compounds and predicting how potential drugs might behave, potentially reducing costs and time. This includes the discovery of entirely new classes of antibiotics.
Understanding AI's Decisions
AI explainability refers to the ability to understand and clearly communicate how an artificially intelligent system makes its decisions or predictions.
There is often a trade-off between AI performance and the ease of explaining its decision-making process. Complex AI models can achieve high performance but are harder to explain, while simpler models are more understandable but may perform less effectively. One method for explaining AI decisions uses a ‘what if’ approach, altering input data to observe changes in the AI’s output. This technique generates heat maps that highlight where the AI is focusing, though not necessarily why it makes specific inferences. Advancing more explainable and interpretable AI models requires collaboration between clinicians and computer scientists.
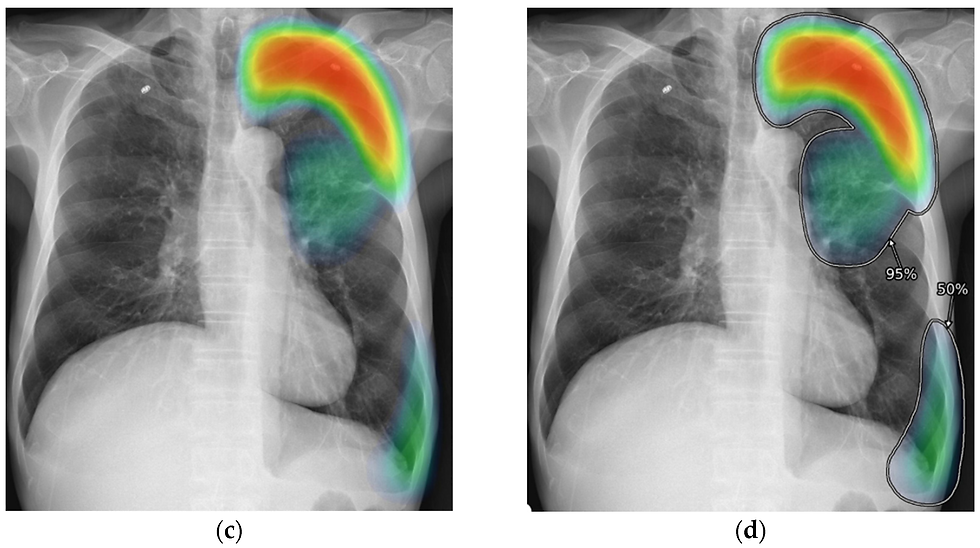
Ethical Challenges
AI in healthcare brings transformative potential, but it also poses significant ethical challenges that must be addressed to ensure its safe and equitable use.
Bias: AI systems are trained on data that may not fully represent the population, leading to bias and inaccurate diagnoses for underrepresented groups—a critical issue requiring urgent attention.
Transparency: AI systems sometimes identify patterns based on irrelevant factors, such as differences in image formats across hospitals, rather than genuine clinical indicators. This raises concerns about transparency and the need to ensure AI learns meaningfully.
Overdiagnosis: While AI can detect conditions at very early stages, such as cancers that are not immediately life-threatening, this can result in unnecessary treatments for patients who may not require them.
Limitations: AI systems have sometimes struggled to perform outside of research settings, such as during the COVID-19 pandemic. Poor-quality data, lack of diversity, and insufficient labelling in training datasets are significant contributing factors.
The Future of AI in Healthcare
The general consensus is that AI will enhance, rather than replace, the decision-making and execution skills of healthcare professionals. AI systems serve as tools to help healthcare providers gain deeper insights and make more informed decisions. The human element of healthcare remains indispensable, as the human touch plays a critical role in supporting patients’ mental well-being during diagnosis and treatment. Multimodal AI is an emerging area of research that seeks to develop systems capable of integrating and interpreting diverse types of healthcare data—such as images, test results, and patient histories—over time, to provide a more holistic view of a patient’s condition and hopefully improve outcomes.
Final Thoughts
AI has immense potential to revolutionise healthcare by improving efficiency, reducing costs, and enabling personalised care. However, addressing ethical challenges is crucial to ensure these systems remain transparent, unbiased, and serve as tools to support, rather than replace, healthcare professionals. The future of AI in healthcare is bright, with innovations in multimodal AI poised to bring unprecedented sophistication to the field.
If you enjoyed reading, don’t forget to subscribe to our newsletter for more, share it with a friend or family member, and let us know your thoughts—whether it’s feedback, future topics, or guest ideas, we’d love to hear from you!
Comments